Hyperparameter Tuning And Its Techniques In Machine Learning Shantha
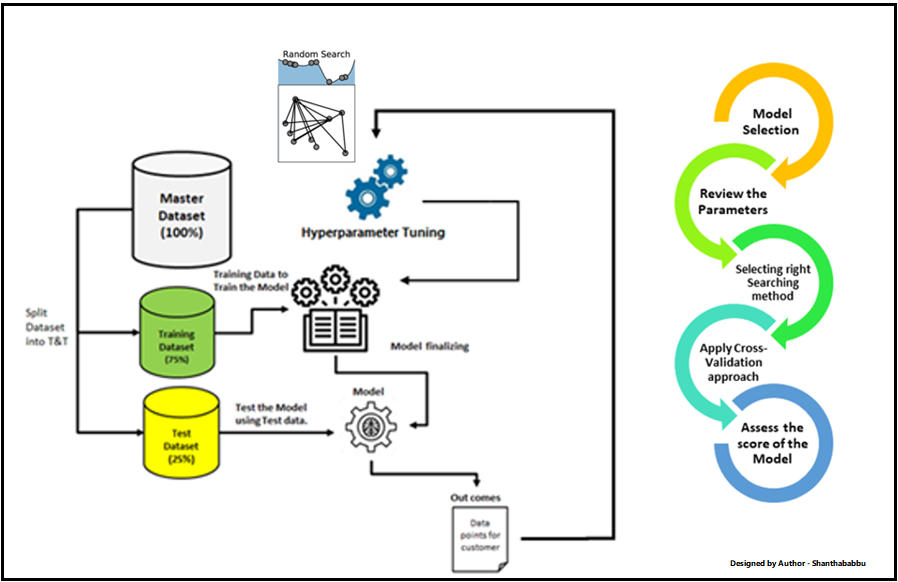
Hyperparameter Tuning And Its Techniques In Machine Learning вђ Shantha Make sure to use k fold while using hyperparameter tuning to improvise your hyperparameter tuning and coverage of hyperparameter space. go with a better combination for hyperparameters and build strong results. i trust, this article helps you to understand the concepts and ways to implement the same. An example of hyperparameter tuning is a grid search. in grid search, the data scientist or machine learning engineer defines a set of hyperparameter values to search over, and the algorithm tries all possible combinations of these values. for example, if the hyperparameters include the learning rate and the number of hidden layers in a neural.
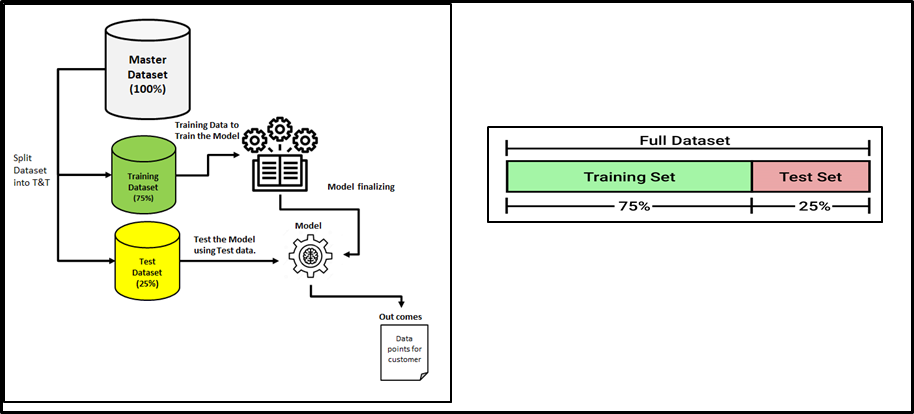
Hyperparameter Tuning And Its Techniques In Machine Learning вђ Shantha Abstract. hyperparameter tuning is essential for optimizing the performance and generalization of machine learning (ml) models. this review explores the critical role of hyperparameter tuning in. Hyperparameter tuning is a final step in the process of applied machine learning before presenting results. you will use the pima indian diabetes dataset. the dataset corresponds to a classification problem on which you need to make predictions on the basis of whether a person is to suffer diabetes given the 8 features in the dataset. Machine learning algorithms have hyperparameters that allow you to tailor the behavior of the algorithm to your specific dataset. hyperparameters are different from parameters, which are the internal coefficients or weights for a model found by the learning algorithm. unlike parameters, hyperparameters are specified by the practitioner when configuring the model. typically, it is challenging […]. Performing k fold cross validation allows us to “improve the estimated performance of a machine learning model” and is typically utilized when performing hyperparameter tuning. the last thing you want when tuning hyperparameters is to run a long experiment on a randomized set of data, obtain high accuracy, and then find the high accuracy.
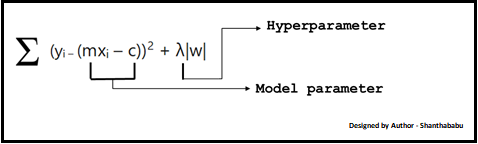
Hyperparameter Tuning And Its Techniques In Machine Learning вђ Shantha Machine learning algorithms have hyperparameters that allow you to tailor the behavior of the algorithm to your specific dataset. hyperparameters are different from parameters, which are the internal coefficients or weights for a model found by the learning algorithm. unlike parameters, hyperparameters are specified by the practitioner when configuring the model. typically, it is challenging […]. Performing k fold cross validation allows us to “improve the estimated performance of a machine learning model” and is typically utilized when performing hyperparameter tuning. the last thing you want when tuning hyperparameters is to run a long experiment on a randomized set of data, obtain high accuracy, and then find the high accuracy. Hyperparameter tuning is a process of selecting the optimal values for hyperparameters of the machine learning model. the values are determined after iterating through different combinations of hyperparameter values with a model and comparing the metrics evaluation results. when coupled with cross validation techniques, this results in training. This book dives into hyperparameter tuning of machine learning models and focuses on what hyperparameters are and how they work. this book discusses different techniques of hyperparameters tuning, from the basics to advanced methods, giving you all you need to optimize your applications.
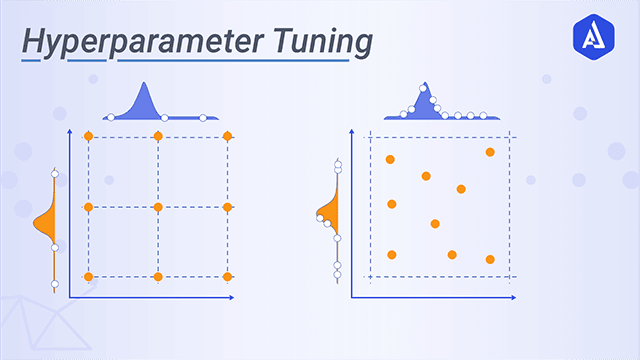
Hyperparameter Tuning For Machine Learning Models Akira Ai Hyperparameter tuning is a process of selecting the optimal values for hyperparameters of the machine learning model. the values are determined after iterating through different combinations of hyperparameter values with a model and comparing the metrics evaluation results. when coupled with cross validation techniques, this results in training. This book dives into hyperparameter tuning of machine learning models and focuses on what hyperparameters are and how they work. this book discusses different techniques of hyperparameters tuning, from the basics to advanced methods, giving you all you need to optimize your applications.
Comments are closed.