Principle Of Least Squares Method

Principle Of Least Squares Method Youtube The method of least squares is a parameter estimation method in regression analysis based on minimizing the sum of the squares of the residuals (a residual being the difference between an observed value and the fitted value provided by a model) made in the results of each individual equation. the most important application is in data fitting. Following are the steps to calculate the least square using the above formulas. step 1: draw a table with 4 columns where the first two columns are for x and y points. step 2: in the next two columns, find xy and (x) 2. step 3: find ∑x, ∑y, ∑xy, and ∑ (x) 2. step 4: find the value of slope m using the above formula.
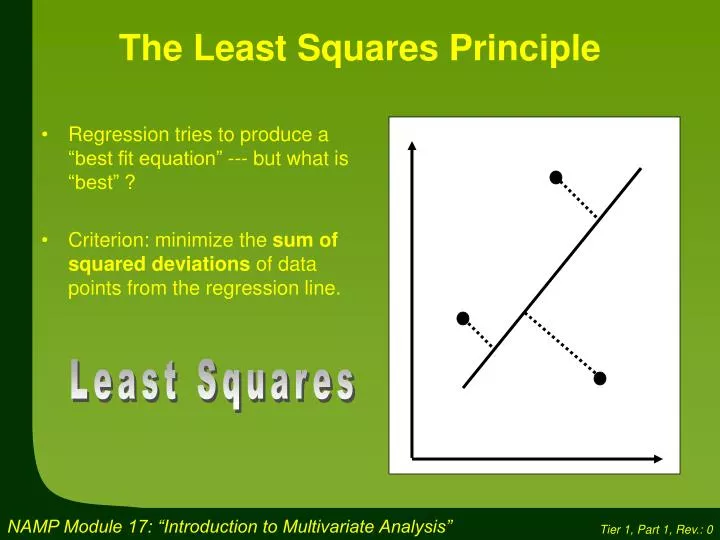
Ppt The Least Squares Principle Powerpoint Presentation Id 1167934 The least squares method is a very beneficial method of curve fitting. despite many benefits, it has a few shortcomings too. one of the main limitations is discussed here. in the process of regression analysis, which utilizes the least square method for curve fitting, it is inevitably assumed that the errors in the independent variable are. Least squares method: the least squares method is a form of mathematical regression analysis that finds the line of best fit for a dataset, providing a visual demonstration of the relationship. 7.3 least squares: the theory. now that we have the idea of least squares behind us, let's make the method more practical by finding a formula for the intercept a 1 and slope b. we learned that in order to find the least squares regression line, we need to minimize the sum of the squared prediction errors, that is: q = ∑ i = 1 n ( y i − y. Least squares principle is a widely used method for obtaining the estimates of the parameters in a statistical model based on observed data. suppose that we have measurements \(y 1,\ldots,y n\) which are noisy versions of known functions \(f 1(\beta),\ldots,f n(\beta)\) of an unknown parameter \(\beta\). this means, we can write.
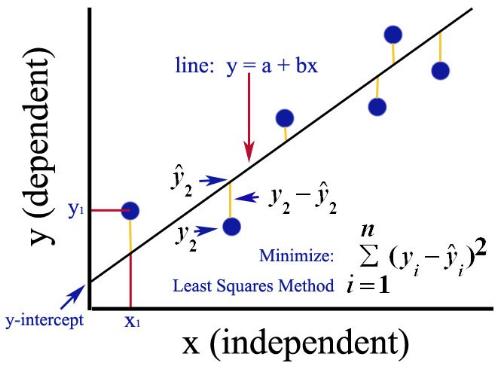
Least Squares Method Techintroduce 7.3 least squares: the theory. now that we have the idea of least squares behind us, let's make the method more practical by finding a formula for the intercept a 1 and slope b. we learned that in order to find the least squares regression line, we need to minimize the sum of the squared prediction errors, that is: q = ∑ i = 1 n ( y i − y. Least squares principle is a widely used method for obtaining the estimates of the parameters in a statistical model based on observed data. suppose that we have measurements \(y 1,\ldots,y n\) which are noisy versions of known functions \(f 1(\beta),\ldots,f n(\beta)\) of an unknown parameter \(\beta\). this means, we can write. Least squares method, in statistics, a method for estimating the true value of some quantity based on a consideration of errors in observations or measurements. in particular, the line (the function yi = a bxi, where xi are the values at which yi is measured and i denotes an individual observation) that minimizes the sum of the squared. 7 limitations of least squares 13 8 least squares in r 14 9 propagation of error, alias \the delta method" 18 1 recapitulation let’s recap from last time. the simple linear regression model is a statistical model for two variables, xand y. we use x| the predictor variable | to try to predict y, the target or response1. the assumptions of the.
:max_bytes(150000):strip_icc()/LeastSquaresMethod-4eec23c588ce45ec9a771f1ce3abaf7f.jpg)
Least Squares Method What It Means How To Use It With Examples Least squares method, in statistics, a method for estimating the true value of some quantity based on a consideration of errors in observations or measurements. in particular, the line (the function yi = a bxi, where xi are the values at which yi is measured and i denotes an individual observation) that minimizes the sum of the squared. 7 limitations of least squares 13 8 least squares in r 14 9 propagation of error, alias \the delta method" 18 1 recapitulation let’s recap from last time. the simple linear regression model is a statistical model for two variables, xand y. we use x| the predictor variable | to try to predict y, the target or response1. the assumptions of the.
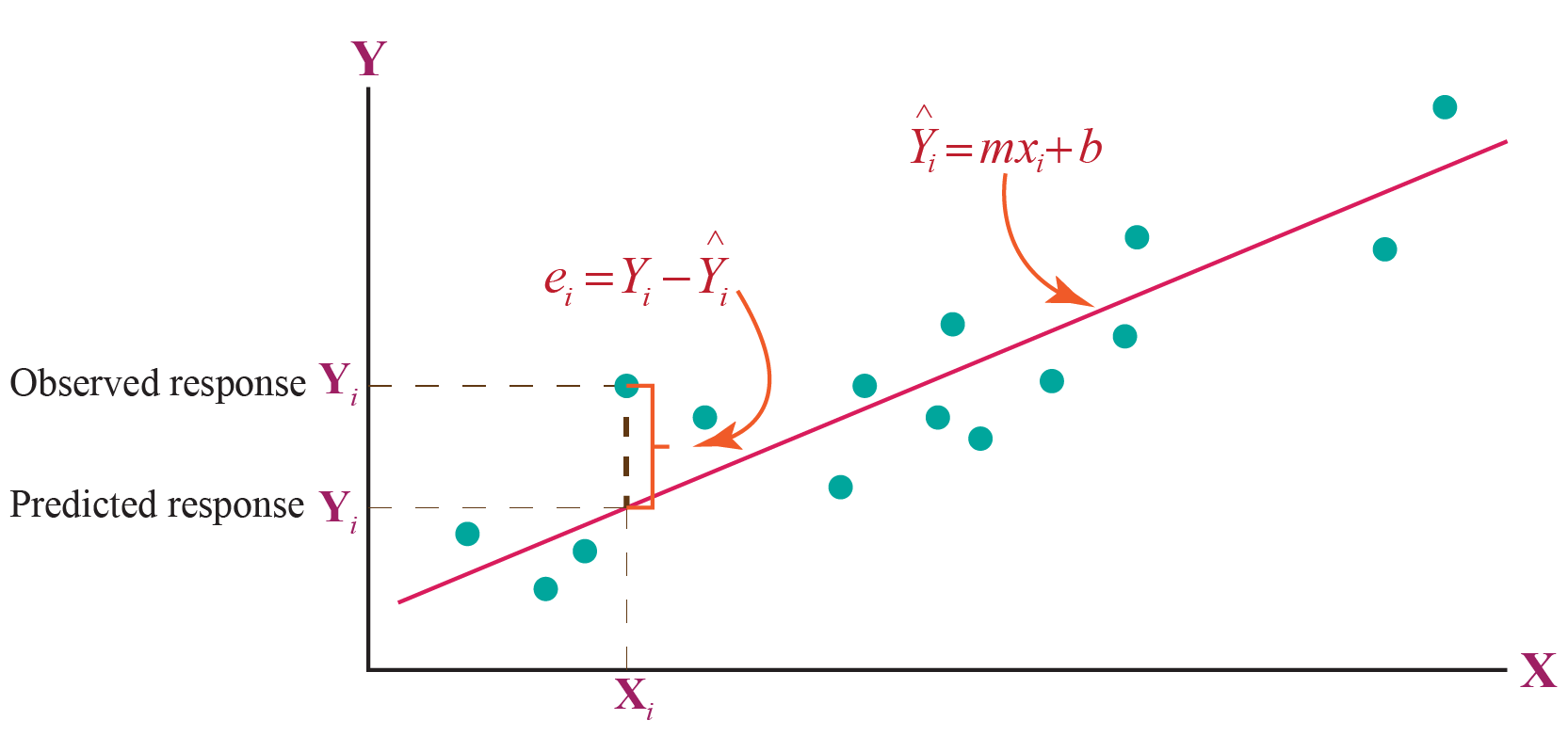
Least Squares Cuemath
Comments are closed.