Statistical Inference Pdf Statistical Inference Bayesian Inference

Statistical Inference 2nd Edition Pdf Ready For Ai • bayesian computation via variational inference. • some subtle issues related to bayesian inference. 12.1 what is bayesian inference? there are two main approaches to statistical machine learning: frequentist (or classical) methods and bayesian methods. most of the methods we have discussed so far are fre quentist. it is important to. Statistical inference is the procedure of drawing conclusions about a population or process based on. a sample. characteristics of a population are known as parameters. the distinctive aspect of. bayesian inference is that both parameters and sample data are treated as random quantities, while. other approaches regard the parameters non random.
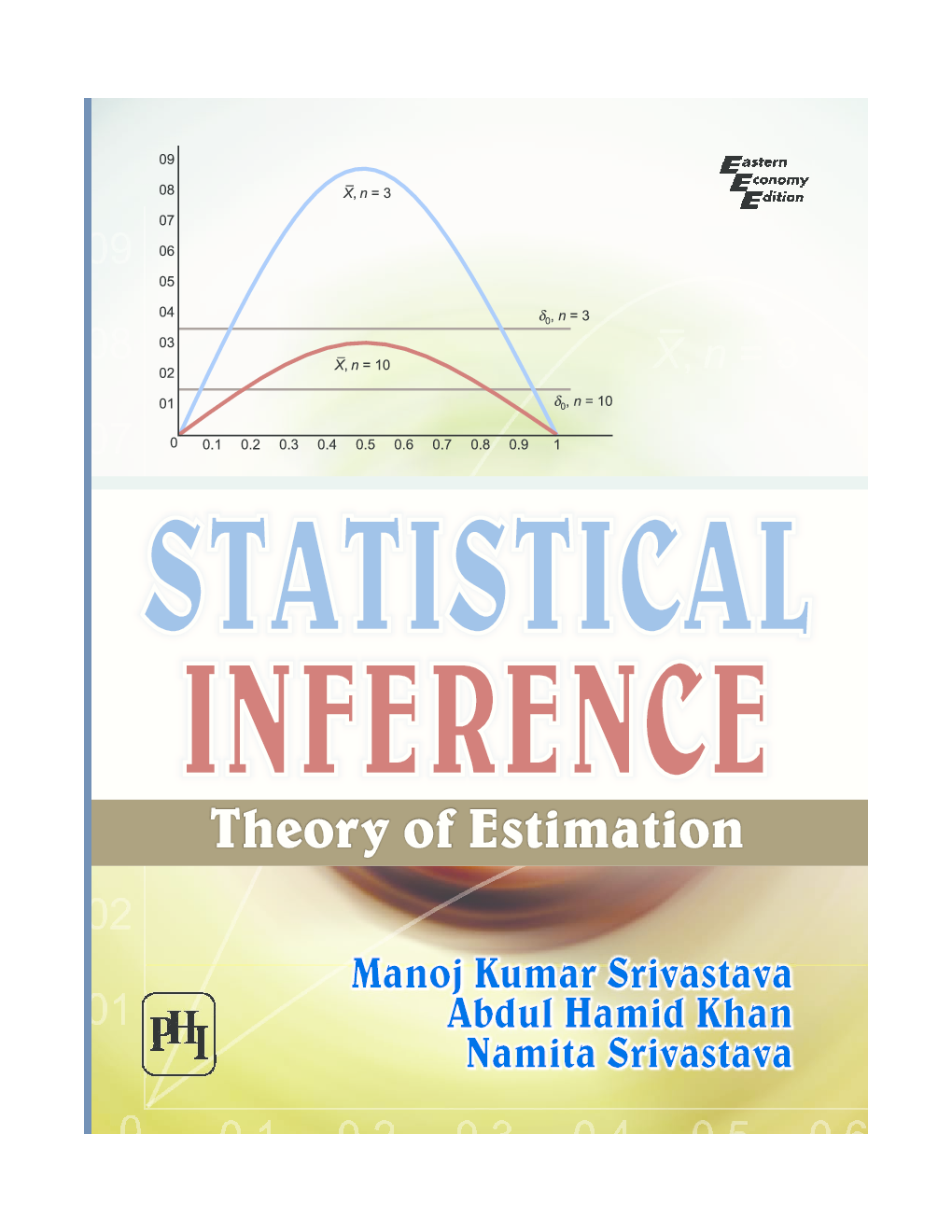
Download Statistical Inference Theory Of Estimation Pdf Online By Abstract | bayesian statistics is an approach to data analysis based on bayes’ theorem, where available knowledge about parameters in a statistical model is updated with the information in observed data. the background knowledge is expressed as a prior distribution, and combined with observational data in the form of a likelihood function to. Abstract. we present basic concepts of bayesian statistical inference. we briefly introduce the bayesian paradigm. we present the conjugate priors; a computational convenient way to quantify prior information for tractable bayesian statistical analysis. we present tools for parametric and predictive inference, and particularly the design of. 1 introduction to bayesian statistical inference 5 bayes (parametric) point estimator of θ ∈ with respect to the loss function (θ , δ) and the posterior distribution π( θ | x 1 : n ) is an. 1.1 statistical inference: motivation statistical inference is concerned with making probabilistic statements about ran dom variables encountered in the analysis of data. examples: means, median, variances example 1.1. a company sells a certain kind of electronic component. the company.
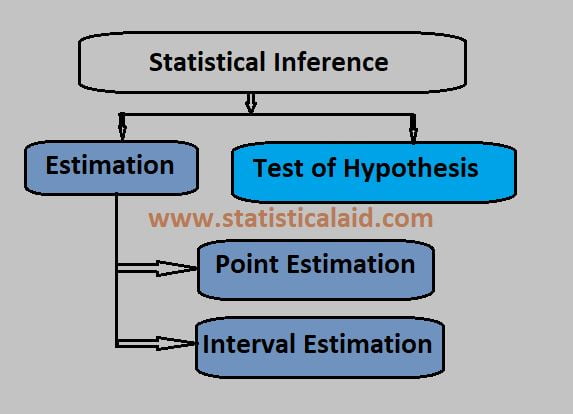
Statistical Inference Books Pdf вђ Collegelearners 1 introduction to bayesian statistical inference 5 bayes (parametric) point estimator of θ ∈ with respect to the loss function (θ , δ) and the posterior distribution π( θ | x 1 : n ) is an. 1.1 statistical inference: motivation statistical inference is concerned with making probabilistic statements about ran dom variables encountered in the analysis of data. examples: means, median, variances example 1.1. a company sells a certain kind of electronic component. the company. In frequentist inference, the unknown parameter is not a random vari able, so talking about its \distribution" makes no sense. even in bayesian inference, the likelihood is still the same mathematical object as the pmf or pdf of the data. hence, it describes probabilities of observing data values given certain parameter values, not the other way. These lecture notes provide a rapid, accessible introduction to bayesian statistical methods. the course covers the fundamental philosophy and principles of bayesian inference, including the reasoning behind the prior likelihood model construction synonymous with bayesian methods, through to advanced topics such as nonparametrics, gaussian processes and latent factor models.
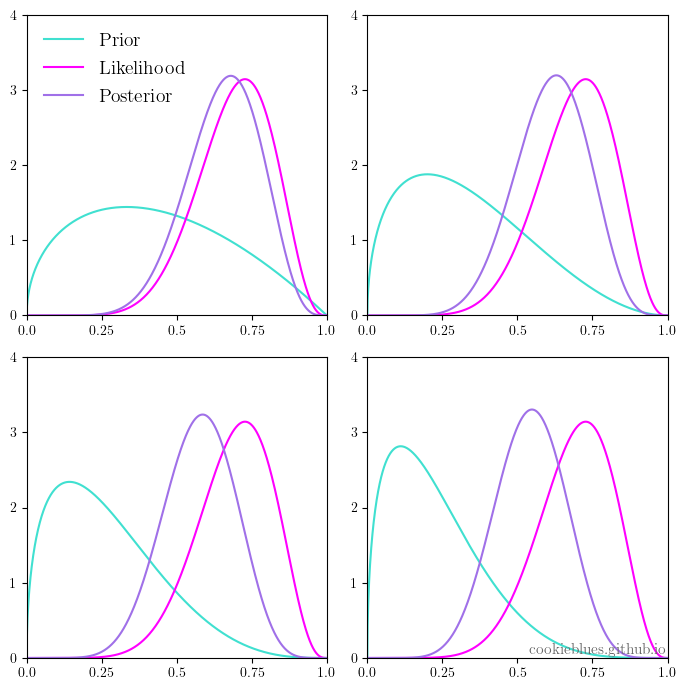
What Is Bayesian Inference Towards Data Science In frequentist inference, the unknown parameter is not a random vari able, so talking about its \distribution" makes no sense. even in bayesian inference, the likelihood is still the same mathematical object as the pmf or pdf of the data. hence, it describes probabilities of observing data values given certain parameter values, not the other way. These lecture notes provide a rapid, accessible introduction to bayesian statistical methods. the course covers the fundamental philosophy and principles of bayesian inference, including the reasoning behind the prior likelihood model construction synonymous with bayesian methods, through to advanced topics such as nonparametrics, gaussian processes and latent factor models.
Comments are closed.